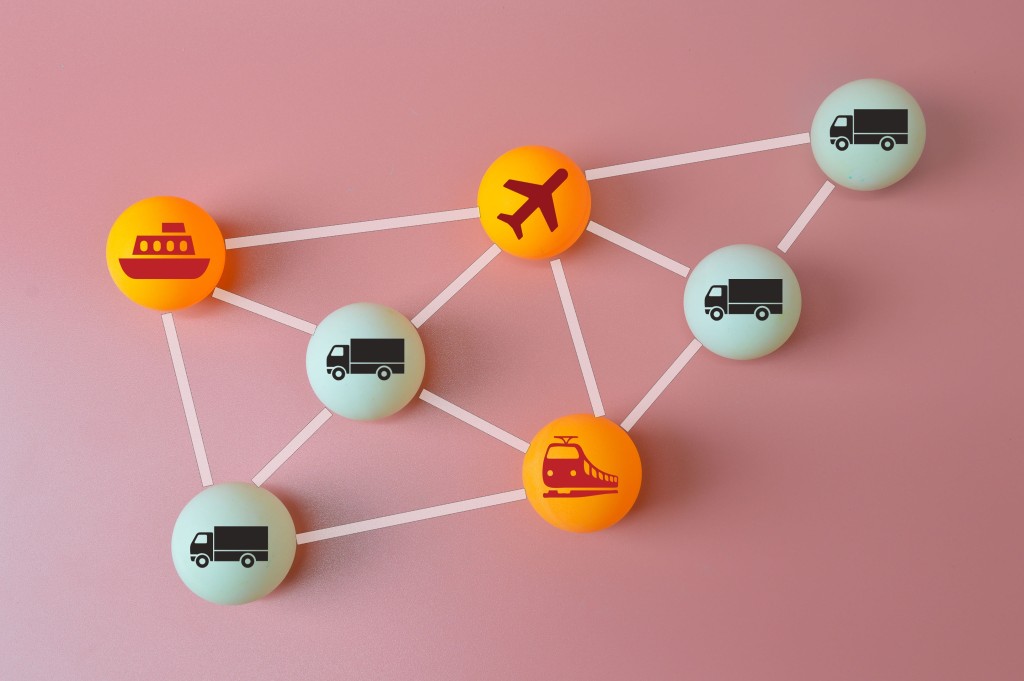
Supply chain management is critical to any business operation, ensuring the smooth flow of goods and services from suppliers to customers. Data science and analytics have emerged as powerful tools for optimizing supply chain processes in recent years. Companies can gain by leveraging large volumes of data and applying advanced analytical techniques.
Valuable insights into their supply chains, identify inefficiencies, and make informed decisions to improve overall performance. This essay will explore examples of how data science and analytics transform supply chain management.
One example of the application of data science in supply chain management is demand forecasting. Accurate demand forecasting is crucial for businesses to optimize inventory levels, production schedules, and distribution strategies. Companies can develop sophisticated predictive models that accurately forecast future demand by analyzing historical sales data and external factors such as economic indicators or weather patterns. These models enable businesses to adjust their operations accordingly, minimizing stockouts or excess inventory.
Another area where data science plays a significant role in supply chain management is logistics optimization. Transportation costs account for a substantial portion of the overall supply chain expenses. Data-driven approaches can help identify the most cost-effective routes, modes of transportation, or even optimal warehouse locations based on factors like customer demand patterns or fuel prices. For instance, companies can use machine learning algorithms to analyze historical shipping data and identify ways that indicate the most efficient routes for specific types of products.
Data science also enables better supplier relationship management by providing insights into supplier performance and risk assessment. Companies can evaluate suppliers objectively and make informed decisions about sourcing strategies by analyzing parameters such as delivery times, quality metrics, or financial stability indicators using advanced analytics techniques like clustering or anomaly detection algorithms.
Furthermore, real-time monitoring using IoT devices and advanced analytics allows businesses to track shipments’ status continuously. This helps identify potential bottlenecks or delays early on so that appropriate actions can be taken promptly to mitigate risks.
Data science has revolutionized inventory management by enabling companies to implement just-in-time (JIT) inventory systems. JIT systems
aim to minimize inventory holding costs while ensuring enough stock is available to meet customer demand. By analyzing historical sales data, market trends, and other relevant factors, businesses can accurately determine the optimal reorder points and quantities for each product, reducing the risk of stockouts or excess inventory.
From demand forecasting to logistics optimization and supplier relationship management, these technologies provide valuable insights that help businesses make informed decisions and improve overall performance. As companies continue to generate vast amounts of data, harnessing its potential through advanced analytical techniques will be crucial for staying competitive in today’s dynamic business environment.